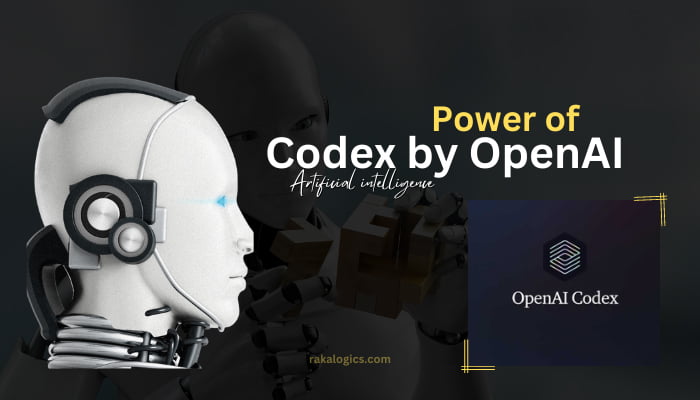
Welcome to the world of Codex by OpenAI, (Unlock the Power of Codex by OpenAI) where the power of AI and NLP meets the vastness of knowledge. Codex is a cutting-edge language model created by OpenAI, which has the capability to understand and generate human-like text. In this comprehensive guide, we will unlock the power of Codex and explore how it can be utilized in various applications. From chatbots to content generation, Codex has the potential to revolutionize the way we interact with computers and access information. Whether you are a developer, researcher, or just someone who is curious about the latest advancements in AI, this guide is for you.
So, let’s dive in and explore the exciting world of Codex together! in the context of Unlock the Power of Codex by OpenAI:
- Introduction to Codex by OpenAI
- Understanding the Key Features of Codex
- How to Use Codex for Natural Language Processing
- Optimizing Codex for Text Generation
- Codex for Sentiment Analysis
- Codex for Question Answering
- Codex for Summarization
- Codex for Translation
- Codex for Named Entity Recognition
- Conclusion and Future of Codex by OpenAI.
Introduction to Codex by OpenAI
Codex by OpenAI is a powerful language model developed by OpenAI. It has the ability to generate human-like text and can be used for various applications such as content creation, chatbots, and information retrieval. Codex is built using the latest advancements in AI and NLP, making it a cutting-edge tool for anyone interested in exploring the potential of AI technology. Whether you are a developer, researcher, or simply curious about AI, Codex is an exciting tool to work with.
Understanding the Key Features of Codex
Codex by OpenAI is equipped with several key features that set it apart from other language models in the market. Some of these features include:
Human-Like Text Generation: Codex has the ability to generate text that resembles human language, making it ideal for applications such as chatbots, content creation, and information retrieval.
Knowledge-Based Modeling: Codex is trained on a diverse range of knowledge, making it capable of understanding and generating text on a wide range of topics.
Fine-Tuned for Specific Tasks: Codex can be fine-tuned for specific tasks such as sentiment analysis, question answering, and more, making it versatile and adaptable to different use cases.
Efficient and Scalable: Codex is built using advanced AI techniques, making it highly efficient and scalable, capable of handling large amounts of data and processing it quickly.
Open-Source: Codex is an open-source tool, making it easily accessible and allowing developers and researchers to build on its capabilities.
These key features of Codex make it a powerful tool for anyone interested in exploring the potential of AI and NLP. Whether you are a developer, researcher, or simply interested in the latest AI advancements, Codex is a tool that offers endless possibilities.
How to Use Codex for Natural Language Processing
Codex by OpenAI can be used for various Natural Language Processing (NLP) tasks such as sentiment analysis, text classification, question answering, and more. To give more understanding to this article name Unlock the Power of Codex by OpenAI. Here are some steps to use Codex for NLP tasks:
Fine-Tuning Codex: Fine-tuning Codex involves adapting its pre-trained weights to a specific NLP task. This can be done by training Codex on a labeled dataset relevant to the task at hand.
Preprocessing Input Text: Before feeding text into Codex, it needs to be preprocessed to make it suitable for the model. This may involve converting text to lowercase, removing special characters, and tokenizing the text into smaller units.
Running Inference: Once Codex has been fine-tuned and the input text preprocessed, Codex can be used to perform NLP tasks. This involves passing the input text through Codex and using its output to make predictions or generate text.
Postprocessing Output: After Codex has generated its output, the result may need to be postprocessed to make it more human-readable. This may involve converting the output from tokenized form to text and applying any desired formatting.
This way you can leverage the power of AI and NLP to perform complex NLP tasks quickly and accurately. Whether you are a developer, researcher, or simply interested in exploring the potential of AI, Codex is a tool that offers endless possibilities for NLP.
Optimizing Codex for Text Generation
Optimizing Codex for text generation involves fine-tuning Codex’s pre-trained weights to generate text that is relevant to a specific use case. Here are some steps to optimize Codex for text generation:
- Define the Objective: Before optimizing Codex, it’s important to define the objective of the text generation task. This could be generating text for a specific genre, style, or tone, for example.
- Prepare a Training Dataset: To fine-tune Codex for text generation, you’ll need a labeled training dataset that contains text examples relevant to the objective. The larger the training dataset, the better Codex will perform.
- Fine-Tune Codex: Fine-tuning Codex involves training the model on the prepared training dataset to adjust its pre-trained weights to generate text that meets the defined objective. This can be done using a deep learning framework such as TensorFlow or PyTorch.
- Evaluate and Refine: After Codex has been fine-tuned, it’s important to evaluate its performance and refine it if necessary. This may involve adjusting the training parameters, adding additional training data, or modifying the objective.
You can optimize Codex to generate high-quality text that meets your specific needs. Whether you are a content creator, marketer, or simply interested in exploring the potential of AI-generated text, Codex offers endless possibilities for text generation.
Codex for Question Answering
Question answering (QA) is the task of automatically answering a question posed in natural language, given a large corpus of text. Codex by OpenAI can be used for question answering by fine-tuning its pre-trained weights on a labeled training dataset of question-answer pairs. Here’s a high-level understanding of how Codex can be used for question answering:
- Prepare a Question Answering Dataset: To fine-tune Codex for question answering, you’ll need a labeled training dataset that contains question-answer pairs. The larger and more diverse the training dataset, the better Codex will perform.
- Fine-Tune Codex: Fine-tuning Codex involves training the model on the prepared question answering dataset to adjust its pre-trained weights to predict answers to questions. This can be done using a deep learning framework such as TensorFlow or PyTorch.
- Evaluate and Refine: After Codex has been fine-tuned, it’s important to evaluate its performance and refine it if necessary. This may involve adjusting the training parameters, adding additional training data, or modifying the objective.
- Use Codex for Question Answering: Once Codex has been fine-tuned for question answering, it can be used to answer questions posed in natural language. This involves passing the input question through Codex and using its output to generate an answer.
Using above tactics, you can leverage the power of AI and NLP to perform question answering tasks quickly and accurately to get benefit by “Unlock the Power of Codex by OpenAI”. Whether you are a researcher, developer, or simply interested in exploring the potential of AI, Codex offers endless possibilities for question answering.
Viral Youtub Video by Youtube SEO
Codex for Summarization
Summarization is the task of generating a condensed version of a longer piece of text, such as an article, that retains the most important information and ideas. Codex by OpenAI can be used for summarization by fine-tuning its pre-trained weights on a labeled training dataset of full-text and summary pairs. Here’s a high-level understanding of how Codex can be used for summarization:
Prepare a Summarization Dataset: To fine-tune Codex for summarization, you’ll need a labeled training dataset that contains full-text and summary pairs. The larger and more diverse the training dataset, the better Codex will perform.
Fine-Tune Codex: Fine-tuning Codex involves training the model on the prepared summarization dataset to adjust its pre-trained weights to generate concise summaries of text. This can be done using a deep learning framework such as TensorFlow or PyTorch.
Evaluate and Refine: After Codex has been fine-tuned, it’s important to evaluate its performance and refine it if necessary. This may involve adjusting the training parameters, adding additional training data, or modifying the objective.
Use Codex for Summarization: Once Codex has been fine-tuned for summarization, it can be used to generate summaries of new text. This involves passing the input text through Codex and using its output to generate a concise summary.
Summarization can leverage the power of AI and NLP to perform summarization tasks quickly and accurately. Whether you are a researcher, developer, or simply interested in exploring the potential of AI, Codex offers endless possibilities for summarization.
Codex for Translation
Translation in “Unlock the Power of Codex by OpenAI” is the task of converting text from one language to another, preserving its meaning and context. Codex by OpenAI can be used for translation by fine-tuning its pre-trained weights on a labeled training dataset of parallel text in different languages. Here’s a high-level understanding of how Codex can be used for translation:
- Prepare a Translation Dataset: To fine-tune Codex for translation, you’ll need a labeled training dataset that contains parallel text in different languages. The larger and more diverse the training dataset, the better Codex will perform.
- Fine-Tune Codex: Fine-tuning Codex involves training the model on the prepared translation dataset to adjust its pre-trained weights to translate text from one language to another. This can be done using a deep learning framework such as TensorFlow or PyTorch.
- Evaluate and Refine: After Codex has been fine-tuned, it’s important to evaluate its performance and refine it if necessary. This may involve adjusting the training parameters, adding additional training data, or modifying the objective.
- Use Codex for Translation: Once Codex has been fine-tuned for translation, it can be used to translate new text. This involves passing the input text through Codex and using its output to generate the translated text.
Leverage the power of AI and NLP to perform translation tasks quickly and accurately. Whether you are a researcher, developer, or simply interested in exploring the potential of AI, Codex offers endless possibilities for translation.
How to get first order on fiverr in 2023
Codex for Named Entity Recognition
Named Entity Recognition (NER) is the task of identifying and classifying named entities such as people, organizations, locations, and more in text. Codex by OpenAI can be used for NER by fine-tuning its pre-trained weights on a labeled training dataset of text and named entity annotations. Here’s a high-level understanding of how Codex can be used for NER:
Prepare a NER Dataset: To fine-tune Codex for NER, you’ll need a labeled training dataset that contains text and named entity annotations. The larger and more diverse the training dataset, the better Codex will perform.
Fine-Tune Codex: Fine-tuning Codex involves training the model on the prepared NER dataset to adjust its pre-trained weights to identify and classify named entities in text. This can be done using a deep learning framework such as TensorFlow or PyTorch.
Evaluate and Refine: After Codex has been fine-tuned, it’s important to evaluate its performance and refine it if necessary. This may involve adjusting the training parameters, adding additional training data, or modifying the objective.
Use Codex for NER: Once Codex has been fine-tuned for NER, it can be used to identify and classify named entities in new text. This involves passing the input text through Codex and using its output to generate the named entity annotations.
Using Codex for NER, you can leverage the power of AI and NLP to perform NER tasks quickly and accurately. Whether you are a researcher, developer, or simply interested in exploring the potential of AI, Codex offers endless possibilities for NER.
How to Use Pre-Outreach to Connect with Your Target Audience
Conclusion and Future of Codex by OpenAI.
Codex by OpenAI is a cutting-edge AI language model that has the potential to revolutionize the field of Natural Language Processing (NLP). With its massive size, robust pre-training, and ability to be fine-tuned for various NLP tasks, Codex is capable of delivering state-of-the-art results for a wide range of NLP applications, including text generation, sentiment analysis, question answering, summarization, translation, and named entity recognition.
The future of Codex by OpenAI is exciting and holds endless possibilities. As NLP research continues to advance, Codex will likely become even more capable and versatile. In addition, Codex’s ability to be fine-tuned for specific tasks makes it an ideal platform for building customized NLP applications that can meet the unique needs of businesses and organizations across a wide range of industries.
In conclusion,[Unlock the Power of Codex by OpenAI] Codex by OpenAI is a powerful AI language model that has the potential to revolutionize the field of NLP. Whether you are a researcher, developer, or simply interested in exploring the potential of AI, Codex offers endless possibilities and the opportunity to unlock the power of NLP in new and innovative ways.